Hello, welcome to this lesson on mastering statistics. We're going to continue working with hypothesis testing. In this particular case, we're going to start talking about the concept of a p-value. Keep in mind that this is in the context of large sample hypothesis testing of means. Our sample size is greater than 30. Up until now, we've been using rejection regions to determine if we should reject the null hypothesis. We set up boundaries based on the level of significance, alpha, and calculate the test statistic from our data to see where it falls. Depending on its position, we can determine if the null hypothesis has been rejected or not. Now, let's get into the topic of p-values. This is something that gives many students a lot of trouble and confusion. However, I am excited because I believe I can explain it to you with concrete examples. After we go through a couple of examples, I'm confident that you will have a very good understanding of what p-values are intuitively. Before diving into the concept, I want you to keep in mind the purpose of a p-value. The process and reason behind it is not much different from what we have been doing before. The ultimate goal is to determine if we should reject the null hypothesis or fail to reject it. We were using rejection regions to make this determination, and now we will use p-values, which may initially appear different but are essentially the same thing. While rejection regions work perfectly fine in statistics, p-values are more commonly used in real research. If you read a research paper in statistics, they will often discuss p-values to explain the results of a study and the rejection or acceptance of the null hypothesis. This is why it is important for us to learn...
Award-winning PDF software
Video instructions and help with filling out and completing Who Form 8815 Calculators
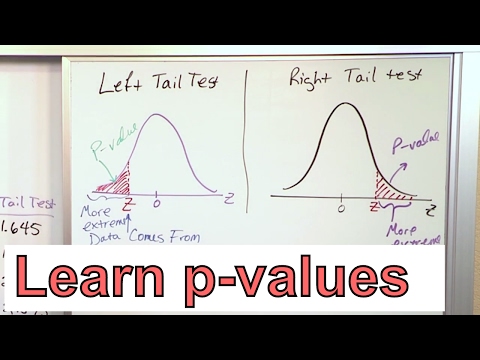